
Supercharging Enterprise AI Pipelines with Specialized Judges
.webp)
"Significant differences in cost appear based on the model chosen and the smaller and/or more specialised models (Veritas and Veritas Nano) are an order of magnitude or more cheaper than the general purpose large language models.”
Matillion's Data Productivity Cloud empowers organizations to build and manage data pipelines faster for AI and analytics at scale. As their enterprise customers increasingly integrated AI into critical workflows, these organizations faced a significant challenge: ensuring the accuracy and reliability of AI-generated outputs without creating bottlenecks in their data pipelines.
To address this growing customer need, Matillion sought a solution that would integrate seamlessly with their platform while providing enterprise-grade evaluation capabilities. That's when they partnered with Collinear to bring specialized AI judge models to their customers.
The Challenge: Empowering Data Pipelines with Reliable AI Evaluation
Matillion customers needed a reliable way to validate AI outputs within their data pipelines and had several critical needs, including:
- Finding the right judge model for diverse use cases — Judge models need to operate across various industries and data types, adding complexity
- Navigating a complex landscape of options — With dozens of potential models from multiple providers, customers struggled to identify which judges would best meet their needs
- Balancing performance with practical constraints — Accuracy alone wasn't enough; evaluation solutions needed to maintain reasonable costs and minimal latency impact on data pipelines
This led Matillion to conduct comprehensive testing of various judge models from leading providers, including Collinear's Veritas solutions, to identify which approaches would best enable their customers to implement reliable AI evaluation without sacrificing pipeline performance or cost efficiency.
The Experiment: Rigorous Model Evaluation
Matillion designed a comprehensive, blinded experiment to identify the most effective AI judge for evaluating AI-generated content. They tested a focused selection of models from leading providers including OpenAI (GPT-4o, GPT-4o-mini) and Anthropic (Claude Sonnet, Claude Haiku). Also included were Collinear's specialized reliability-focused judges Veritas and Veritas Nano.
The experiment used real-world consumer electronics reviews, evaluating each model's ability to detect when another AI had incorrectly classified whether a review contained product defect information.
Their methodical approach compared model performance across both zero-shot (no examples) and few-shot (with examples) scenarios, measuring precision, recall, and F1 scores to determine which solution would deliver the most reliable results for their customers' data pipelines.

The Solution: Specialized AI Judges Deliver Superior Results
The results revealed a clear winner: Collinear's specialized Veritas judges dramatically outperformed general-purpose alternatives in real world testing.
- Veritas achieved a remarkable 96% F1 score with perfect precision and 92% recall As Matillion noted, "With a few examples, the specialised model really shines for this use case."
- Even Veritas Nano reached 93% F1, outperforming much larger models while requiring significantly fewer resources
- General-purpose models showed inconsistent performance, with some actually declining in effectiveness when given examples
.webp)

This decisive performance gap demonstrated that purpose-built evaluation models deliver superior results for enterprise applications—allowing Matillion's customers to implement reliable AI evaluation without sacrificing pipeline performance or cost-efficiency.
The Impact: Transforming AI Pipeline Quality
By integrating Collinear's Veritas into their workflows, Matillion empowered their customers to:
- Validate AI outputs automatically with enterprise-grade accuracy
- Reduce operating costs while maintaining superior quality standards
- Iterate rapidly on AI implementation strategies without waiting for manual reviews
- Monitor production models continuously to ensure persistent quality
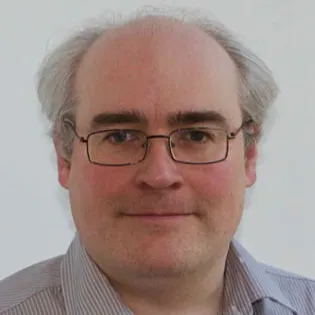
"Significant differences in cost appear based on the model chosen and the smaller and/or more specialised models (Veritas and Veritas Nano) are an order of magnitude or more cheaper than the general purpose large language models.”
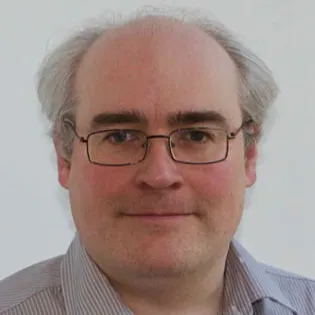
For Matillion's customers, this means confidently deploying AI within their data workflows, knowing that dedicated AI judges are continuously ensuring output quality without creating operational bottlenecks or excessive costs.
Collinear's Veritas has proven to be the optimal solution for enterprise-grade reliability detection, delivering superior performance while maintaining reasonable operational costs—exactly what teams need to scale AI with confidence.
Ready to transform your AI safety strategy?
Don't let outdated evaluations leave your enterprise vulnerable. Experience the future of continuous, proactive AI safety with Collinear.
Take a look at one of our CollinearSafe assessment reports to uncover AI model weaknesses through our proprietary CollinearSafe red-teaming.
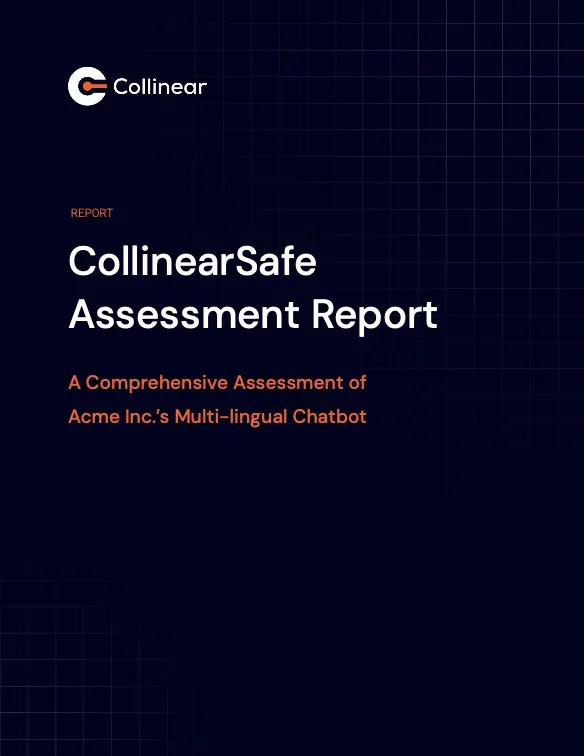
Schedule a live demo today to see how you can secure your AI solution to get to production faster.
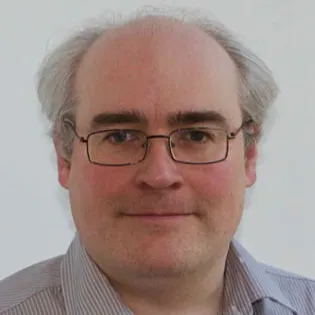